Table of Content
28 December 2015, Volume 23 Issue 12
Journal Introduction
Started in 1982(Monthly)
Editor-in-Chief:FEI Weiyang
Executive Editor-in-Chief: LUO Guangsheng
Sponsored: Chemical Industry and Engineering Society of China,
Chemical Industry Press Co., Ltd.
ISSN: 1004-9541
CN: 11-3270/TQ
Editor-in-Chief:FEI Weiyang
Executive Editor-in-Chief: LUO Guangsheng
Sponsored: Chemical Industry and Engineering Society of China,
Chemical Industry Press Co., Ltd.
ISSN: 1004-9541
CN: 11-3270/TQ
Editorial NewsMore...
Promotion
Most DownloadMore...
InformationMore...
LinksMore...
- Chemical Industry and Engineering Society of China
- Chemical Industry Press Co., Ltd.
- China Association for Science and Technology
- Chinese Academy of Sciences
- Chinese Academy of Engineering
- OSID
- CNKI
- Wanfang Data
- Scopus
- ScienceDirect
- Clarivate Analytics
- CIESC Journal
- Chemical Industry and Engineering Progress
- Energy Storage Science and Technology
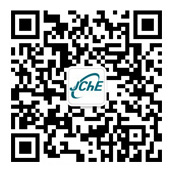
CJCHE Wechat
AdvertisementMore...